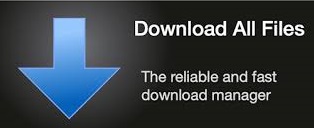

Calvin's agents individually implement high level abstractions of parts of the information retrieval task, integrating information from disparate sources. Calvin observes users while they are accessing documents, proac- tively finds related documents, and provides a unified interface to the informa- tion environment. This paper summarizes how motivations and issues for a multi- agent approach to information retrieval are addressed in our multi-agent system called Calvin. The enormous amount of information available electronically, com- bined with the wide range of methods for information retrieval and manipulation, produces a complex electronic information environment that may be difficult for users to exploit. In addition, we describe a deep convolutional neural network that demonstrates satisfactory performance on this query formulation task when evaluated on the publicly available Avocado dataset and a proprietary dataset of internal emails obtained through an employee participation program. We also present a novel strategy for generating labels from an email corpus-without the need for manual annotations-that can be used to train and evaluate the query formulation model. The query is submitted to an existing IR system to retrieve relevant items for attachment. As email search systems are commonly available, we constrain the recommendation task to formulating effective search queries from the context of the conversations. In this paper, we propose a weakly supervised learning framework for recommending attachable items to the user. A modern email client can proactively retrieve relevant attachable items from the user's past emails based on the context of the current conversation, and recommend them for inclusion, to reduce the time and effort involved in composing the response. Analysis of an enterprise email corpus reveals that 35% of the time when users include these items as part of their response, the attachable item is already present in their inbox or sent folder. More specifically, we propose (1) methods to formulate an effective query from complex textual structures and (2) latent vector space models that circumvent the vocabulary gap in information retrieval.Įmail responses often contain items-such as a file or a hyperlink to an external document-that are attached to or included inline in the body of the message. It is the alleviation of the effect brought forward by this vocabulary gap that is the topic of this dissertation. Consequently, there exists a vocabulary gap between queries and documents that occurs when both use different words to describe the same concepts. However, it is known that a high matching degree at the term level does not necessarily mean high relevance and, vice versa, documents that match null query terms may still be relevant. Inversely, term-based approaches assume documents that do not contain query terms as irrelevant. While term-based approaches are intuitive and effective in practice, they are based on the hypothesis that documents that exactly contain the query terms are highly relevant regardless of query semantics. When presented with a search query, the engine then ranks documents according to their relevance scores by computing, among other things, the matching degrees between query and document terms. Text-a document or a query-is represented by a bag of its words that ignores grammar and word order, but retains word frequency counts. Search engines rely heavily on term-based approaches that represent queries and documents as bags of words.
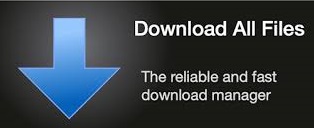